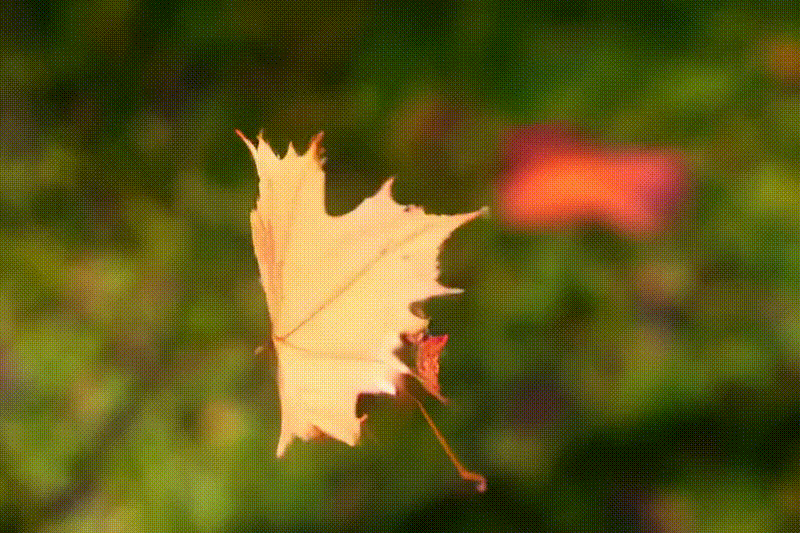
MatchDiffusion: Training-free Generation of Match-Cuts
Alejandro Pardo*, Fabio Pizzati*, Tong Zhang, Alexander Pondaven, Philip Torr, Juan Camilo Perez, Bernard Ghanem
Under Review - Project Page / arXiv / Code
We introduce a training-free method for generating match-cuts using text-to-video diffusion models. By leveraging the denoising process, our approach creates visually coherent video pairs with shared structure but distinct semantics, enabling the creation of seamless and impactful transitions.